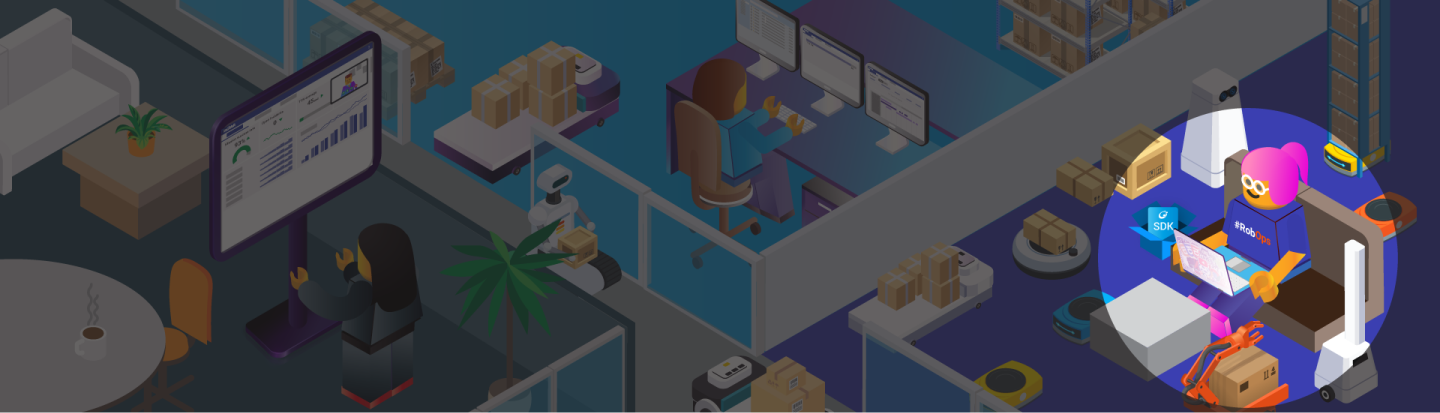
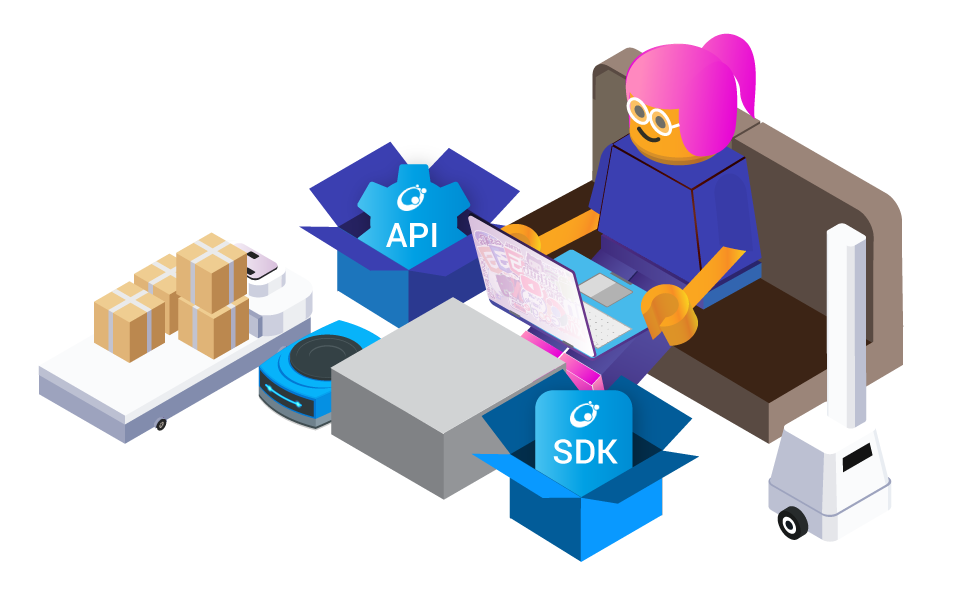
.png?width=295&height=216&name=ipad%20robot%20db%201%20(1).png)
Optimize your data volume so that you only review what you really need to see in secure environments with constrained connectivity.
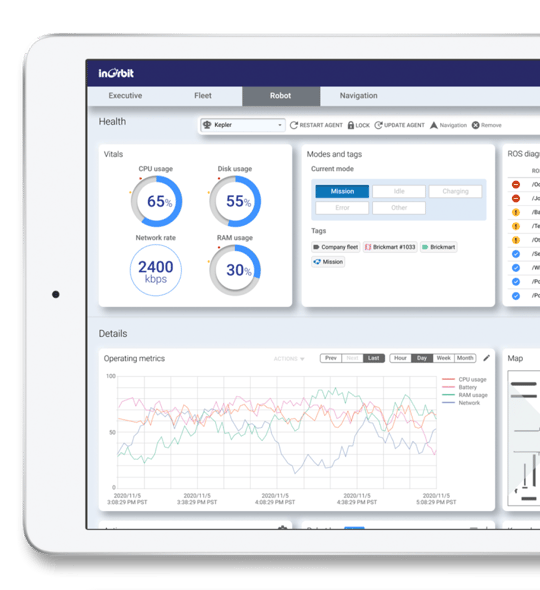
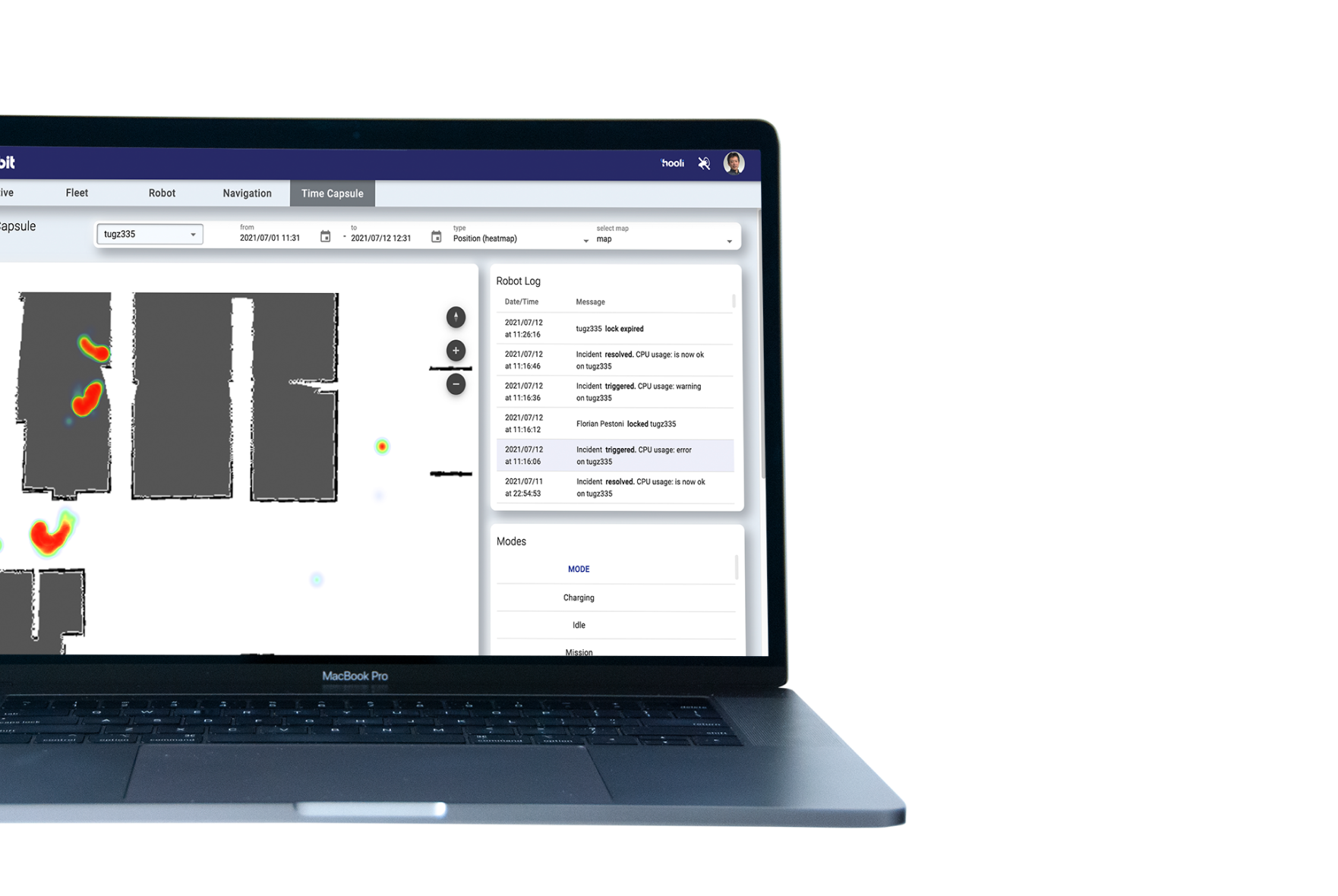
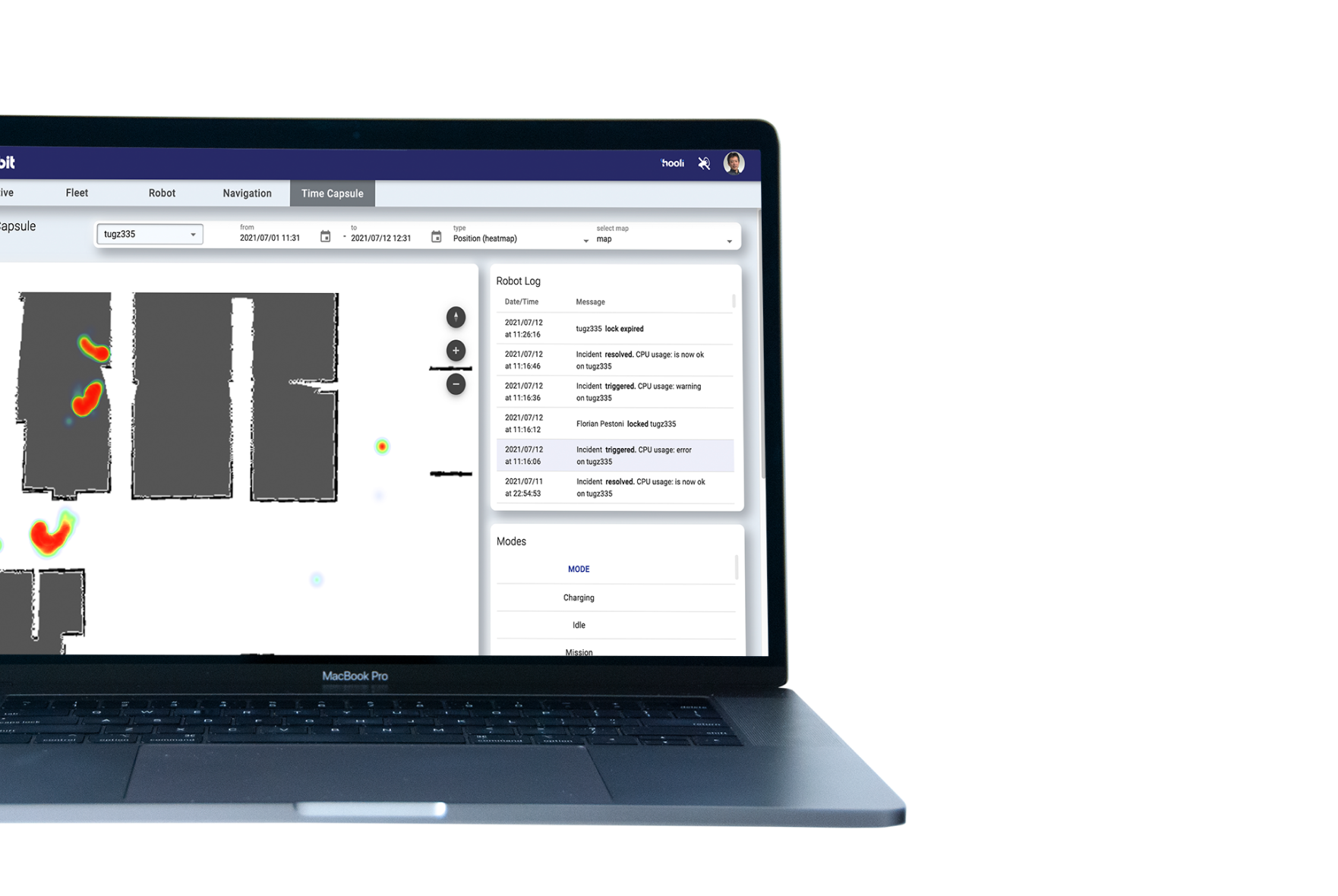
Arm yourself with the relevant information to effectively and confidently determine the sources of problems that required your team’s attention.
.png)
Track and learn from the patterns emerging around repetitive failures that can consume your team’s resources then quickly adapt your system to minimize or avoid them entirely.
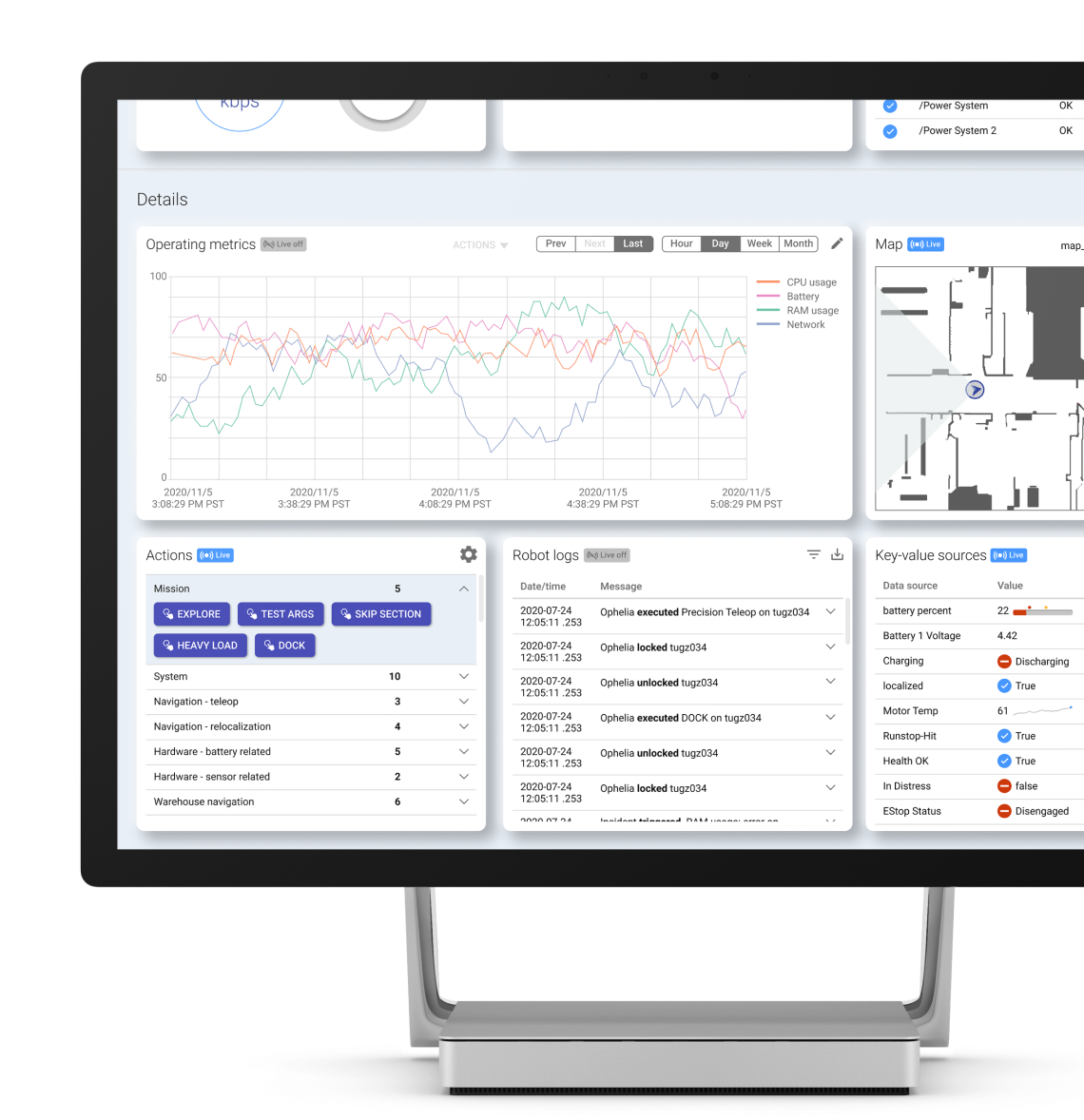
Enabling direct SSH (secure shell) access to robots may be OK in the lab, but exposes robots in the field to unnecessary risks, such as password sharing, cut-and-paste errors for custom commands, etc. Some robotics deployments may rely on VPN (virtual private network) connections to achieve a degree of security, but this often results in connectivity issues.
InOrbit handles end-to-end security for all data communication between its agent and the cloud, establishing a secure, reliable, bi-directional channel with advanced key management. In addition, data is protected on the wire and at rest, using dedicated cryptographic keys.
Robotics developers must learn from failure situations and edge cases to improve the degree of autonomy and reduce the need for remote interventions. However, the tools that work in the lab do not necessarily work for a distributed fleet. Using InOrbit Time Capsule and Data Backfill capabilities, roboticists and software developers can benefit from cloud access to detailed robot data and personalized views that let them drill down to individual robot features, perform easy retroactive analysis, and identify the root cause of a problem.
In order for robots to continuously improve their efficiency, their software must evolve in response to new data. New software versions must be matched to specific hardware. In addition to its core algorithms, each individual robot may require specific information to operate in a given environment, such as maps or schedules, which may change frequently. As part of advancing the state of the art in RobOps, InOrbit enables configuration management best practices, allowing companies to remotely update the configuration and software on the robot over the air, either individually or through configurable collections.
Scaling a robot fleet requires continuous learning from patterns and edge cases emerging in the field. InOrbit’s agent runs on each robot to optimize data collection, analysis at the edge, and transmission to the cloud for further processing. Leveraging InOrbit’s Adaptive Diagnostics allows the agent to adjust the data sampling rate, resolution and summarization in real time, adapting to specific needs across the fleet.
Leveraging the abundant data from a variety of robot data sources, InOrbit can apply artificial intelligence and machine learning techniques to analyze patterns to generate actionable insights and recommendations that translate into cost savings through efficiencies. This can help companies anticipate problems before they occur.
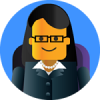

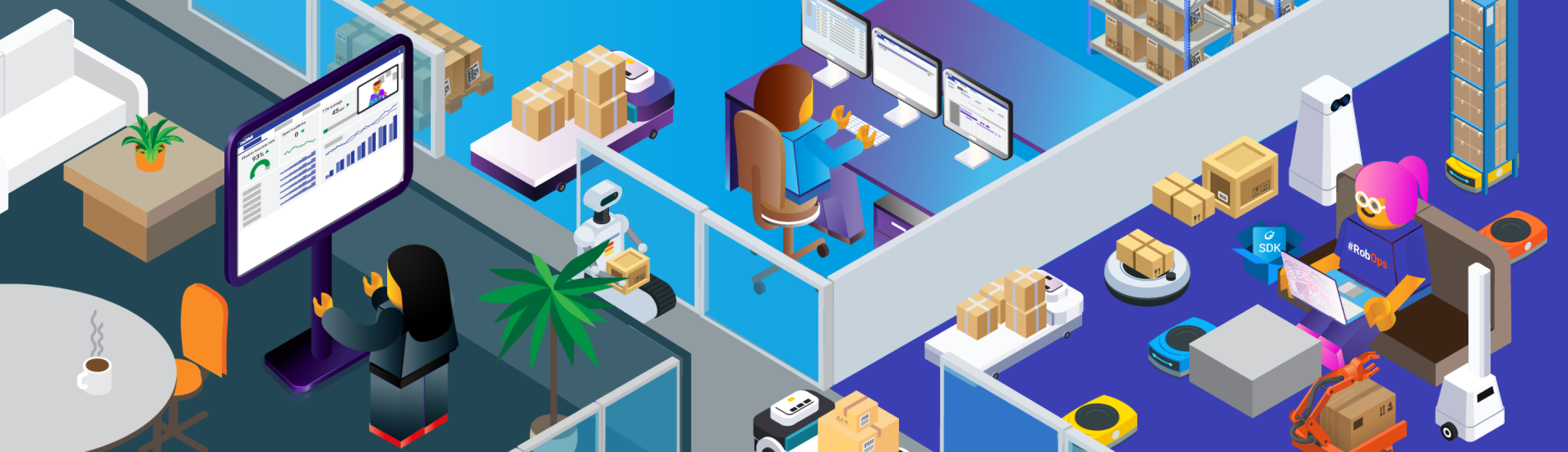